INCRMNTAL is NOT an MMM platform.
I would like to start this post by saying this, as we often get miscategorized as an “MMM” and just as often, people do not understand how different it is what INCRMNTAL does vs. Media Mix Modeling.
In the following article, I will explain what MMM can’t do, that INCRMNTAL can, and vice versa, what MMM can do that INCRMNTAL does not do.
If you’re new here: What is MMM ?
Media Mix Modeling or MMM in short, is a method used to evaluate the effectiveness of different advertising activities over results. MMM uses a top down econometrics approach to find correlations between the mix of channels and results, in order to predict future results – hence the name Media Mix Modeling.
How is INCRMNTAL similar to MMM ?
Both INCRMNTAL and MMM utilize aggregated data (non PII data)
Both INCRMNTAL and (modern) MMM use machine learning and predictive models
Both INCRMNTAL and MMM are used to evaluate advertising effectiveness
The similarities end here.
INCRMNTAL vs. MMM: What can’t MMM do ?
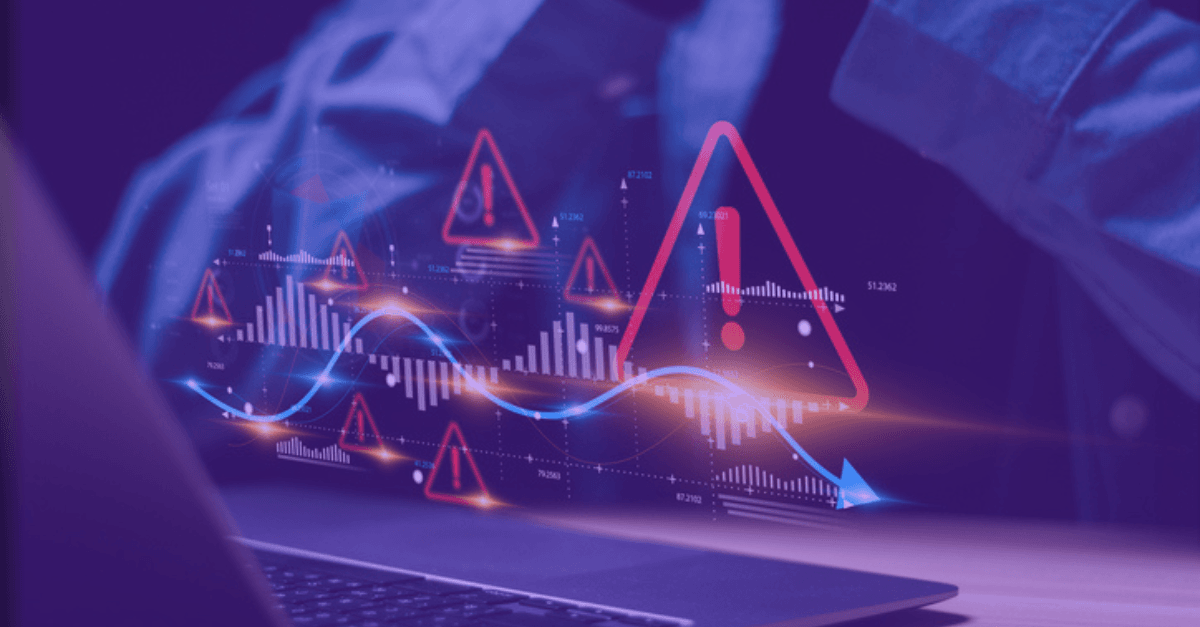
- Ch Ch-ch-ch-ch-changes!
MMM isn’t good at handling an environment where there are multiple changes happening to various campaigns around the same time period. For an MMM to function well, it would require advertisers to try and isolate changes, so that MMM models can identify clear correlations between changes and impact.
INCRMNTAL actually relies and assumes that advertisers make changes, and a lot of them. The modeling process is a bottom up process, relying on the tiniest of changes: creative changes, bid changes, spend changes, campaigns pause / start / decrease / increase. Skeptics might ask: “but what if we don’t make a lot of changes?”. For those, we just refer them to their own Google Ads change history, or Meta Ads change log and you’ll be surprised by the number of changes you make within just one week.
One of the main reasons MMM has not gained widespread adoption, despite the recent hype surrounding the topic, is its requirement to isolate changes.
- Testing a new channel shouldn’t be a sacrifice
Media Mix Modeling trains on past data. Whatever wasn’t seen in the past, can’t be included in the model. When testing a new channel, running it for 1 week, for example, would represent only 2% of the training period (1/52). Testing a new channel using an MMM requires you to spend a significant amount of time, and a significant portion of your budget, for MMM to be able to compute the correlations, and assign a β.
Testing several new channels is not recommended with MMM. For the average fast moving performance marketer – this limitation is a key reason why MMM may not be the most suitable approach. INCRMNTAL shines in measuring a new channel, within days after an advertiser starts it. Even if it represents less than 1% of spend.
- Siamese Channels
If you started two channels, or two campaigns at the same time, and made no changes to these – an MMM would assign the same value to both, even if one of those channels has an ad spend of $100 per day, and the other - $1,000 per day. MMM uses correlations between spending patterns and performance to split the performance across the various elements of the marketing mix.
INCRMNTAL can split the distribution based on any historical data from the channel, or any variance, even the smallest. This is because INCRMNTAL measures “bottom up”
- Granularity? Forget about it!
MMM doesn’t get to campaign level, and for advertisers that do not have significant ad spend across all channels, MMM often does not even get into channel level, but instead, would rely on bundling channels into “Search” (Google, Apple Search Ads), “Social” (Meta, TikTok, Snapchat, Reddit), “Video” (YouTube, Roku, Vibe), etc…
MMM will have significant challenges with DSPs, ad networks, influencers, and any channels where performance may not be consistent, or spend might run in bursts.
INCRMNTAL goes all the way to campaign level, acknowledging creative changes as well, and it shines at measuring omni-channels, and influencers.
- Introducing: BIAS!
MMM requires priors and posteriors to be set (i.e. “what do you believe the ROAS of Apple Search Ads is?”)
Priors and Posteriors can also be “hacked” by running an incrementality measurement.
So – MMM requires incrementality to function.
Incrementality, and INCRMNTAL – does not need MMM.
INCRMNTAL does not require or rely on any priors. The only calibration it allows is a data calibration:
Inclusion of external variables (self-selected by the model if the model finds relevancy)
Including missing data (missing ad spends)
Including missing significant activities (promotions, issues)
MMM vs. INCRMNTAL: What can it do better ?
The primary goal of an MMM is to allow advertisers to assess their high level channel performance, and run planning scenarios such as:
- What would happen to CPA if we increased spend by 30%?
- If we shifted $1M spend from Meta to Google – what could it do to profits?
- What could be a good mix of ad spend if we want to reduce spend by 30%?
MMM is an awesome playground for a CMO or CFO, but it loses its prestige once advertisers try using it to make more granular decisions such as:
- What is the value of a new channel?
- Which campaign should we increase spend on?
- Which campaigns or channels are cannibalizing organics?
- What’s the value of last week’s app featuring?
- What’s the value of the promotion we ran?
In Summary
MMM, like Incrementality, is here to stay. The methodology may be celebrating its 65th birthday, but is still relevant today as a planning tool.
Where MMM failed was over-promising and under delivering. MMM solutions pitched their technology as the next “attribution” platform. Yet, this can’t be further from the truth.
Please note that INCRMNTAL does not think that MMM is “bad”. MMM has some valid use-cases that it is great for. We actually wrote a highly regarded whitepaper on the topic of how to make attribution, incrementality, and MMM work well together. You can find it here: Measurement Orchestration
If you are thinking of adopting an orchestration approach, reach out to us, and we would love to geek out measurement with you!